INTRODUCTION
There are several approaches related to health care management and management indicators based on processes in hospital institutions; they can be summarized in two main visions: clinical (clinical management) and administrative (hospital processes management)1.
Hospital facilities play a predominant role within the National Health System. They are characterized by providing specialized medical care and preventive, curative and rehabilitative nursing care on an uninterrupted basis to all their patients, in addition to providing inpatient, outpatient and emergency services.
Healthcare has evolved from the traditional view towards a more active participation of the patient in the care process. This new approach enhances the participation of patients and accompanying persons in the management, as well as in the development of health services2. In addition, the need is recognized that health services, like any other type of entity, must be properly planned, organized, directed and controlled3,4.
Thus, it is recognized how improvements to hospital management guarantee that standardization results in the optimization of care, product of the transformation of inputs into output as in itself is the welfare of people1, through an integrated, systematic and efficient management, which offers sustained functionality, supported by scientific and technological advances, according to the planned goals and purposes5.
In hospitals there is evidence of movement of patients through a variety of departments, facilities and physical locations at any time, to be attended by different specialists and technicians; this movement is recognized in literature as patient flow. Dawoodbhoy et al6. define it as the ability of healthcare systems to manage patients care demands efficiently by reducing waiting times between treatment stages and average length of stay, while maintaining service levels and patient satisfaction at all times.
Organizing patient flows represents a major challenge for administrators, as medical activity has inherently high variability, which makes it very complex. Thus, identifying the origins of the flows is emerging as an element of great importance that supports decision making. Duarte Forero and Camacho Oliveros7 state that they are generated through the outpatient department (outpatient consultation), emergencies (on-call department), or referrals from other health institution.
Improving patient flow along the process is a long-term work that requires synchronization between all the processes involved. A basic step for its improvement is to identify the points where the flow is interrupted, sequences of activities and limited resources conditioning proactive capacity planning8. Different modeling tools have been developed to represent scenarios for decision making and to approach path-based management through a holistic perspective. Their selection will be in correspondence with basic aspects, such as multifaceted nature, uncertainty and flows complexity.
There are different methods to patient flow modeling: analytical approaches (queuing theory models, Markov chains and compartmental models), simulation-based approaches (discrete event simulation, agent-based simulation and system dynamics), and statistical or empirical approaches9. Their main objectives are capacity planning, resources allocation, resources and appointments scheduling, care processes change, and reducing patient delays.
Markov chains are stochastic processes with finite countable state space, where there is a loss of memory of past states, since only the position at the current instant is taken into account to know the probability of a change of state or not in the chain10. It takes into account parameters such as service time, waiting time, absorption state and phase type distribution. They have been applied to model the length of stay of patients in inpatient and surgical wards11,12.
Those tools have evolved along time, accompanied by the level of hospital processes digitalization, supporting decision making. Their use for patient flows management is still focused on the occupancy of bed resource than on the trajectory. A previous bibliographic search revealed that there were no bibliometric studies showing the evolution of Markov chains application to improvement of patient flows in hospital institutions.
Consequently, the present research aims to evaluate the scientific activity related to the application of Markov chains in the improvement of patient flows in hospital institutions.
METHODS
This is a descriptive-retrospective and observational bibliometric study. The collection of documents was carried out through the ScienceDirect website (https://www.sciencedirect.com/), due to its large number specialized in health field, in addition to allowing parameterized searches based on the combination of descriptors in the title, abstractor and keywords13. The search for information was carried out on January 7, 2023; DeCS/MeSH terms selected were: “hospitals”, “management”, “Markov chain and patient flows”, restricted only to research in English, by identifying in a first review that the main journals specialized in the subject are Anglo-Saxon and that the results obtained from the Spanish search were null; the descriptors are related by using the Boolean operator AND, from which the following search strategy was defined:
EB1: “Markov chain” AND “hospitals”.
EB2: “Markov chain” AND “hospitals” AND “management”.
EB3: “Markov chain” AND “hospitals” AND “management” AND “patient flows”.
The graphic 1 description of the methodology to be implemented, and the identification of specific objectives.
For overall analysis of trends in the evolution of Markov chains application to hospital institutions (EB1), their specific use in hospital management (EB2), and in the improvement of patient flows (EB3), the bibliometric indicator productivity (number of articles per year obtained) was used for the period 1999-2023, analysis that is complemented by the trends in the periods 2019-2023 (last five years) and 2021-2023 (last three years); they were represented after eliminating duplicate documents, and were in the order of 520, 331 and 9 respectively for EB1, EB2 and EB3. The objective of the strategies is to evidence the trend of Markov chains applications first in hospital environments up to their use for the management of patient flows, as the specific field of study of this analysis.
For the specific analysis, only the EB3 strategy is considered according to the objective of the research and the indicators were selected by typology and area of knowledge during the period 1999-2023, to observe the evolution of methodological strategies over time.
While the indicators: quartile, country, number of citations, h-index, Impact Factor (IF), SCImago Journal Rank (SJR) (https://www.scimagojr.com/), CiteScore 2020 and thematic co-occurrence analysis and evolution are applied to the period 2019-2023, being indicators that present a more variable behavior for analysis over long periods of time. For the metadata analysis, three “.RIS” files were downloaded (one per strategy) which were exported and analyzed with the help of the EndNote X8 bibliographic manager (https://es.freedownloadmanager.org/Windows-PC/EndNote.html), and processed in the VOSviewer software (https://www.vosviewer.com/download), to obtain the bibliometric network maps and identify the main research trends.
For the study of bibliometric networks, we take into account Zetty Arenas et al.14 who propose that for the study of co-occurrence of words, each trend is composed of a series of keywords. For validation, a working session is held with one or more experts in the area of knowledge and the consistency of the results with the development and perspective of the area is verified; if the search result is valid, it is documented; otherwise, the search results are considered invalid.
RESULTS
Table 1 shows the descriptive data obtained from the articles found by search strategy. The distribution of papers was analyzed in two main periods: 2019-2023 (last five years) and 2021-2023 (last three years).
There is a trend towards the application of Markov chain analysis in the management of hospital institutions with a representation of 41.6% in the papers of the last five years and 45% in the modeling of patient flows, a trend that increases in the period of the last three years with respect to the last five years in the articles of Markov chains applied in the modeling and management of hospital institutions, while it decreases in the applications focused on the improvement of patient flows.
Table 1 Number of articles retrieved by search strategy

*Percentage of total articles; **Percentage with respect to the total number of articles published in the last five years.
The trend in the period 1999-2023 (graphic 2) shows an increase in scientific publications; however, there is a higher proportion in strategies 1 (EB1) and 2 (EB2).
Graphic 3 shows the number of articles published by type related to patient flow modeling in hospital institutions. It is obtained from the sum of the number of publications per year in the period 1999-2023; data shown in the trend analysis.
Research articles predominated, representing 87.91% of the scientific production (80), followed by review articles representing 4.4% of the scientific production. It should be noted that from 2021 to 2023 the scientific production of research articles increased by 6.11%, which gave greater weight to hospital resource planning based on the application of Markov chain analysis in the modeling of patient flows.
An analysis of the number of articles published by areas of knowledge is made (graph. 4); the most representative are: decision sciences with 53 research articles which represent 58.24%, followed by studies of Medicine and Stomatology which represent 41.76% and those of Computer Sciences represent 40.66%. The main studies from different areas focus on improving patient flows in hospital emergency departments through capacity planning and resource allocation decisions15,16.
An analysis of the journals with more than 2 published articles (table 2), shows that 85.71%6 were located in quartile 1 (Q1), and 14.29% in quartile 2 (Q2). The journals with the highest production were: European Journal of Operational Research (7 articles), Omega (4 articles) and Operations Research for Health Care (4 articles), while Omega had the highest impact with an index of 11.5 in CiteScore 2020, of 2.5 SCImago Journal Rank and an average number of citations per publication of 18.25.
Table 2 Scientific Journal with the highest production on the subject
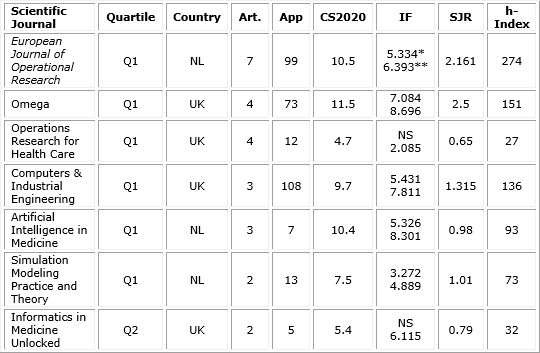
NL: Netherlands; UK: United Kingdom; Art: Number of articles; App: Appointments; CS2020: CiteScore 2020; *IF Web of Science (WoS); **IF SCOPUS; NS: is not shown.
Graphic 5 shows the thematic concurrence network; researches are grouped into four main clusters differentiated by colors:
Green color: Consisting of five main items representing 22.73% of the total number of items: literature review, emergency department, length of stay, patient flow and process mining.
Blue color: Consisting of five main items representing 22.73% of the total number of items: intensive Care Unit (ICU), queuing theory, hospital system, operating room, operating room scheduling, heuristics and capacity.
Red color: Consisting of six main items representing 27.27% of the total number of items: machine learning, efficiency, hospital, simulation and healthcare system.
Yellow color: Consisting of four main items representing 18.18% of the total number of items: Markov chains, healthcare coordination, and optimization.
From the cluster analysis, it is possible to synthesize the main lines of research around the applications of Markov chains in the improvement of patient flows in hospital environments; the information extracted from the articles (objectives and main elements treated or observations) is summarized in chart 17-22.
The main applications of Markov chains can be summarized from the analysis:
1. As a function of resource and patient allocation during treatment stages or trajectory phases,
2. In terms of reducing the length of stay of patients in hospital system, from the perspective of optimizing the sequence of activities that minimize waiting time and case mix study during intra-hospital transfers.
3. Based on the modeling of patient flows in the hospital entity for capacity decision making.
DISCUSSION
Bibliometric studies acquire special relevance among researchers in terms of making contributions to the state of the art and practice in different areas of knowledge, from the identification of trends, lines, impacts and research gaps14. There is an increasing trend in the application of Markov chains in the hospital setting to solve multiple objectives: optimization of care costs11, bed resource allocation18, modeling patient flows21,23, and patient sequencing and coordination19.
Applications in the hospital environment present a growing trend from the consolidation of modeling strategy and process control to the creation of scenarios. In correspondence with the research Sánchez Suárez et al.4 highlights how the assimilation of those tools by health managers becomes a competitive advantage for the health organization.
The predominance of original articles or research is in correspondence with the editorial policies of the impact journals where the studies are reviewed; on the other hand, the research is mainly based on decision science and medicine, in support of the decision-making process18 and process planning21.
The main journals are indexed in SCOPUS, in quartile 1 (Q1), led by the European Journal of Operational Research with the largest number of articles, coinciding with the results obtained. Zetty Arenas et al.14 conducted a study in the SCOPUS database and Web of Science, the main research lines in the thematic industrial automation.
Bibliometric maps point out to the main thematic clusters around applications of Markov chains to management improvement of patient flows in hospital institutions; it is underpinned by the need of a more effective management5 and also reaching a competitive advantage3. However, the possibility of integrating the tool with other modeling and health operations management tools is identified as a gap. Zehrouni et al.21 integrate it with the simulation of discrete events to model complex patient flows in a hospital emergency department, although they do not take into account the demand in this planning, nor the composition of cases into homogeneous groups to simplify management.
The present study didn´t cover analyses of co-authorship, most publishing institutions, collaboration relationships and productivity trends by country, and since it is limited to the English language, its generalization to other languages is an opportunity. On the other hand, the methodology used does not consider other highly impact databases, such as SCOPUS and Web of Science. These issues are recognized as limitations and hence possible future studies in the research topic.
The main applications of Markov chains included resource allocation, reduction of length of stay and flow modeling. This study reveals critical perspectives on the need to integrate modeling tools, in addition to focusing the analysis on patient trajectories or routes to ensure the satisfaction of patients and their companions.
Positive implications of the present research for researchers and health administrators could be mentioned: identification of good practices and tools for modeling and improving patient flows in hospital institutions; identification of gaps, as well as contextualization of possible adjustments to methodologies according to specific conditions; and it lays the foundations for the development of capacity planning procedures based on the optimization of resources through the use of Markovian models. Future research should focus on collaborative analysis, country-specific productivity and generalization to other international impact databases.