Introduction
The current coronavirus disease 2019 (COVID-19) pandemic has been caused by a new, previously unknown severe acute respiratory syndrome coronavirus 2 (SARS-CoV-2). After almost a year of intensive research, there is not yet an efficacious treatment for the disease, and by November 2020 the pandemic seems like gone out of control. Meanwhile, old and new approaches have been tested, and new knowledge is being obtained.
Almost 30 years ago, Professor Irena Cosic proposed the Resonant Recognition Model (RRM) for protein-protein interactions 1-20. Evidence suggests that this approach can shape new therapeutic interventions for a large number of diseases and conditions 4-7.
An area where the RRM could find practical applications is the study of infectious diseases, where interaction between proteins from the infecting agent and receptor proteins from host cells play a key role. Thus RRM has been applied to the study of VIH [8], Plasmodium falciparum 9),(10, Ebola 11 and Zika 12 viruses. Recently, Cosic et al showed that Covid-19 spike S1 proteins might interact with both ACE2 receptors from lung epithelium, as well as Band-3 proteins from human erythrocytes, shading new light into early findings about vascular implications of the disease 13.
This study is also devoted to the RRM analysis of SARS-CoV-2 proteins and their possible interaction with other human proteins.
We focused our study on SARS CoV replicases and methyl transferases, which play a fundamental role in viral replication, and tested, via RRM analysis, for possible interactions with host CD4 T receptor proteins and prohibitins which participate in human organism response to viral infections.
Methods and Materials
Methods-Resonant Recognition Model (RRM)
The Resonant Recognition Model (RRM) is a biophysical model that arises from the postulation that proteins can recognize each other via resonant exchange of electromagnetic energy at very specific frequencies, which are unique for each biological function/interaction 1-3.
These frequencies can be worked out via finding specific periodicities (frequencies) in the distribution of free electron energy along proteins. For finding RRM frequencies, a consensus spectrum is obtained via cross-multiplication of power spectra of protein groups sharing a common function or putatively interacting.
RRM allows performing a detailed analysis of interactions between proteins and putative ligands/targets without any exploration of their homology, 3D structures or complicated physical calculations.
In particular, a key point in the RRM is the demonstration by Cosic that interacting proteins have opposite phases at their characteristic RRM frequency. A phase difference of or about 3.14 rad is considered opposite phase 9), (12), (13. A practical consequence for this is that once the characteristic biological function of the protein’s functional group has been identified, it is possible to design new proteins with the desired frequency components and, consequently, with the desired biological functions 5),(6.
One interesting corollary of RRM approach is the prediction of electromagnetic frequencies at which relevant interactions are taking place. 14
RRM approach has already been successfully applied and experimentally tested in several scenarios 1-15.
Details of RRM procedure have been described in previous publications 15 briefly, in a protein sequence each amino-acid is substituted by its electron-ion - interaction potential value (EIIP), and the obtained succession is treated as a time series. The series is transformed into time domain and relevant frequencies are found via cross multiplication of corresponding spectra. Pairwise comparisons allow finding phase shifts for checking possible protein-protein interactions.
Materials
Protein Sequences Analyzed by RRM
The following protein sequences from UniProt Database have been analyzed using the RRM:
Twenty Human SARS coronavirus methyltransferase proteins:
Q19QX2: 2'-O-methyltransferase - Human SARS coronavirus (SARS-CoV)
A0A6C0X332: orf1ab - 2'-O-methyltransferase - Severe acute respiratory syndrome coronavirus 2 (2019-nCoV)
A0A6B9UY63: orf1ab - 2'-O-methyltransferase - Severe acute respiratory syndrome coronavirus 2 (2019-nCoV)
A0A6C0R287: orf1ab - 2'-O-methyltransferase - Severe acute respiratory syndrome coronavirus 2 (2019-nCoV)
A0A6C0R294: orf1ab - 2'-O-methyltransferase - Severe acute respiratory syndrome coronavirus 2 (2019-nCoV)
A0A679G4B7: orf1ab - 2'-O-methyltransferase - Severe acute respiratory syndrome coronavirus 2 (2019-nCoV)
A0A6C0N6C5: orf1ab - 2'-O-methyltransferase - Severe acute respiratory syndrome coronavirus 2 (2019-nCoV)
A0A679G4C7: orf1ab - 2'-O-methyltransferase - Severe acute respiratory syndrome coronavirus 2 (2019-nCoV)
A0A6C1BAC9: orf1ab - 2'-O-methyltransferase - Severe acute respiratory syndrome coronavirus 2 (2019-nCoV)
A0A6C0N6E9: orf1ab - 2'-O-methyltransferase - Severe acute respiratory syndrome coronavirus 2 (2019-nCoV)
A0A6B9WIH5: orf1ab - 2'-O-methyltransferase - Severe acute respiratory syndrome coronavirus 2 (2019-nCoV)
A0A6B9WIK4: orf1ab - 2'-O-methyltransferase - Severe acute respiratory syndrome coronavirus 2 (2019-nCoV)
A0A6C0QEL8: orf1ab - 2'-O-methyltransferase - Severe acute respiratory syndrome coronavirus 2 (2019-nCoV)
A0A6B9VNL0: orf1ab - 2'-O-methyltransferase - Severe acute respiratory syndrome coronavirus 2 (2019-nCoV)
A0A6C0QEM7: orf1ab - 2'-O-methyltransferase - Severe acute respiratory syndrome coronavirus 2 (2019-nCoV)
A0A6C0QEN3: orf1ab - 2'-O-methyltransferase - Severe acute respiratory syndrome coronavirus 2 (2019-nCoV)
A0A6B9W0R7: orf1ab - 2'-O-methyltransferase - Severe acute respiratory syndrome coronavirus 2 (2019-nCoV)
A0A6B9VSU5: orf1ab - 2'-O-methyltransferase - Severe acute respiratory syndrome coronavirus 2 (2019-nCoV)
A0A6B9VSV5: orf1ab - 2'-O-methyltransferase - Severe acute respiratory syndrome coronavirus 2 (2019-nCoV)
A0A6C0RS15: orf1ab - 2'-O-methyltransferase precursor - Severe acute respiratory syndrome coronavirus 2 (2019-nCoV)
Eight replicase proteins
P0C6F8: Replicase polyprotein 1a - Bat coronavirus HKU3 (BtCoV)(SARS-like coronavirus HKU3)
P0C6T7: Replicase polyprotein 1a - Bat coronavirus Rp3/2004 (BtCoV/Rp3/2004)(SARS-like coronavirus Rp3)
P0C6V9: Replicase polyprotein 1ab - Bat coronavirus 279/2005 (BtCoV)(BtCoV/279/2005)
P0C6W2: Replicase polyprotein 1ab - Bat coronavirus HKU3 (BtCoV)(SARS-like coronavirus HKU3)
P0C6W6: Replicase polyprotein 1ab - Bat coronavirus Rp3/2004 (BtCoV/Rp3/2004)(SARS-like coronavirus Rp3)
P0C6X7: Replicase polyprotein 1ab - Human SARS coronavirus (SARS-CoV)(Severe acute respiratory syndrome coronavirus)
P0DTD1: Replicase polyprotein 1ab - Severe acute respiratory syndrome coronavirus 2 (2019-nCoV)(SARS-CoV-2)
P0C6C1: Replicase polyprotein 1a - Severe acute respiratory syndrome coronavirus 2 (2019-nCoV)(SARS-CoV-2)
Twenty-one prohibitin proteins
G1M116: PHB - Prohibitin - Ailuropoda melanoleuca (Giant panda)
H0UTX5: PHB - Prohibitin - Cavia porcellus (Guinea pig)
A0A3Q2H5B0: PHB - Prohibitin - Equus caballus (Horse)
A8WA76: PHB - Prohibitin - Felis catus (Cat)
U3JWZ8: PHB - Prohibitin - Ficedula albicollis (Collared flycatcher)
P35232: PHB - Prohibitin - Homo sapiens (Human)
F7HLX2: PHB - Prohibitin - Macaca mulatta (Rhesus macaque)
G1MU38: PHB - Prohibitin - Meleagris gallopavo (Wild turkey)
P86220: PHB - Prohibitin - Mesocricetus auratus (Golden hamster)
M3YVB1: PHB - Prohibitin - Mustela putorius furo (European domestic ferret)
K7F9Z0: PHB - Prohibitin - Pelodiscus sinensis (Chinese softshell turtle)
P67779: Phb - Prohibitin - Rattus norvegicus (Rat)
G3VR15: PHB - Prohibitin - Sarcophilus harrisii (Tasmanian devil)
F2Z543: PHB - Prohibitin - Sus scrofa (Pig)
P40961: PHB - Prohibitin - Saccharomyces cerevisiae
P50093: PHB2 - Prohibitin - Caenorhabditis elegans
Q3V235: PHB2 - Prohibitin - Mus musculus(Mouse)
Q2HJ97: PHB2 - Prohibitin - Bos Taurus(Bovine)
Q99623:PHB2 - Prohibitin - Homo Sapiens(Human)
O35129: PHB2 - Prohibitin - Mus musculus(Mouse)
Q5RB19:PHB2- Prohibitin - Pongo abelii(Sumatran orangutan)
Eleven CD4 -T-cell surface antigen T4 proteins
A0A2K5K7H1: CD4 - T-cell surface antigen T4/Leu-3 - Colobus angolensis palliatus (Peters' Angolan colobus)
F6Y6X8: CD4 - T-cell surface antigen T4/Leu-3 - Equus caballus (Horse)
G3QL31: CD4 - T-cell surface antigen T4/Leu-3 - Gorilla gorilla gorilla (Western lowland gorilla)
A0A2K6DWJ0: CD4 - T-cell surface antigen T4/Leu-3 - Macaca nemestrina (Pig-tailed macaque)
I6LI22: CD4 - T-cell surface antigen T4/Leu-3 - Mustela putorius furo (European domestic ferret)
A0A096MWA4: CD4 - T-cell surface antigen T4/Leu-3 - Papio anubis (Olive baboon)
P01730: CD4 - T-cell surface glycoprotein CD4 precursor - Homo sapiens (Human)
P16003: CD4 - T-cell surface glycoprotein CD4 precursor - Macaca mulatta (Rhesus macaque)
P06332: Cd4 - T-cell surface glycoprotein CD4 precursor - Mus musculus (Mouse)
P16004: CD4 - T-cell surface glycoprotein CD4 precursor - Pan troglodytes (Chimpanzee)
P05540: Cd4 - T-cell surface glycoprotein CD4 precursor - Rattus norvegicus (Rat)
Results
SARS CoV Proteins Replicases
In figure 1, the consensus spectrum for coronavirus replicases is shown. A prominent peak appeared at f=0.2839 (corresponding to 708 nm, visible red light).
A much smaller, but detectable second peak appeared at f=0.07439 (Fig. 1a)
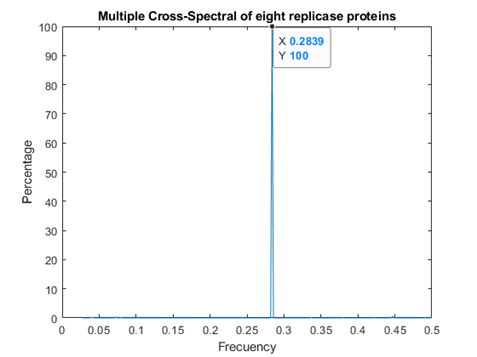
Fig.1 Resonant Recognition Model (RRM) consensus spectrum for SARS-CoV replicases with common RRM characteristic frequencies of f=0.2839. These correspond to wavelengths of 708 nm corresponding to visible red light.
Methyl transferases
In figure 2, the consensus spectrum for methyl transferases is shown. A peak is obtained at f=0.07349 (2735nm, in the infrared region). A smaller, but still observable peak is at f=0.2839, thus coincident with both peaks for replicases.
Consensus Spectrum for Replicases and Methyl Transferases.
In figure 3, it can be observed the presence of both above mentioned frequencies.
Interaction Between Human and SARS Covid-2 Proteins
SARS CoV-Methyl transferases
As figures 4 and 5 revealed, Methyl transferases share common peaks with both prohibitins and CD4 receptors, at f=0.07459. However, opposite phases were observed only for SARS Covid2 methyl transferase and human prohibitin, but not for CD4 (Fig. 6 and 7)
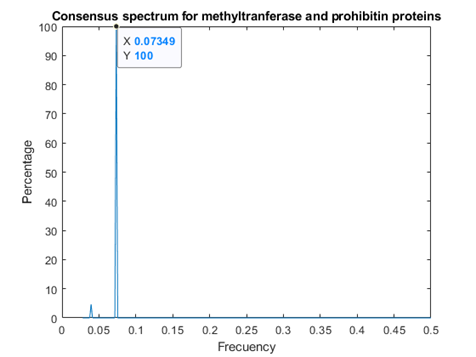
Fig.4 Recognition Model (RRM) consensus spectrum for SARS-CoV-2 methyl transferases and prohibitins with common RRM characteristic frequencies of 0.07349. This corresponds to a wavelength of 2735nm, in the infrared range.
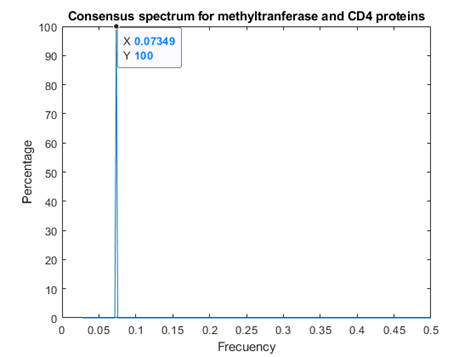
Fig. 5 Resonant Recognition Model (RRM) consensus spectrum for SARS-CoV-2 methyl transferases and CD4 -T-cell surface antigens T4 proteins with common RRM characteristic frequencies of 0.07349.
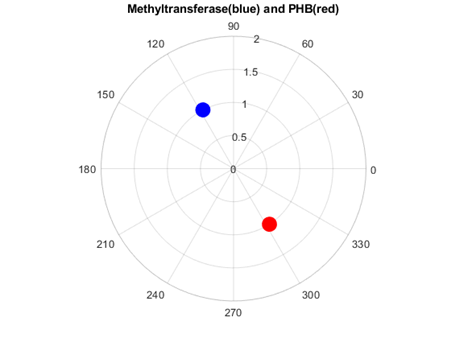
Fig. 6 Phase circles at frequency of 0.07349 for 2'-O-methyltransferase from Severe acute respiratory syndrome coronavirus 2 (SARS‐CoV‐2) with phase of 117.5o in blue, and for human Prohibitin with phase of 302.8o in red. It can be observed that corresponding phases are almost opposite (174.7o or 0.97π rad), supporting the RRM approach that interacting proteins should have opposite phases at frequencies characterizing their recognition and interaction.
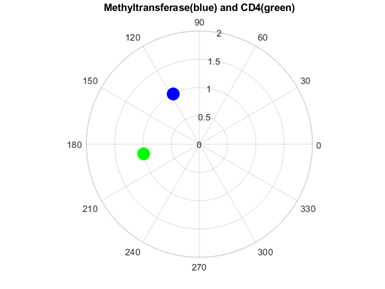
Fig. 7 Phase circles at frequency of 0.07349 for 2'-O-methyltransferase from Severe acute respiratory syndrome coronavirus 2 (SARS‐CoV‐2) with phase of 117.5o in blue, and for human CD4 - T-cell surface glycoprotein precursor with phase of 189.9o in green. It can be easily observed that corresponding phases are far from opposite (72.4o or 0.40π rad phase shift), not lending support for possible interaction.
Replicases
RRM analysis revealed opposite phases at f=0.07349 for SARS Coid2 replicase and Human CD4 T receptor protein (phase shift =1.06π).
Rather different was the interaction of replicases and prohibitins. The consensus spectrum exhibited a peak at f=0.0318 (6321 nm, in the infrared range). At this frequency, opposite phases between SARS Cov2 replicase and human prohibitin was observed (Fig. 8).
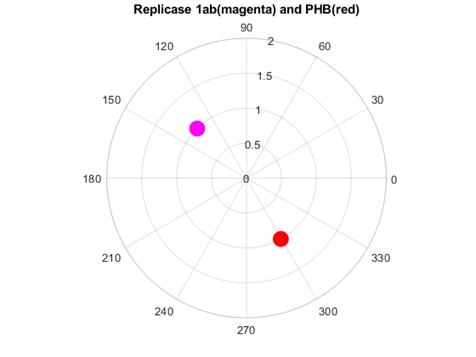
Fig. 8 Phase circles at frequency of f=0.0318 for 2'-O-methyltransferase from Severe Acute Respiratory Syndrome Coronavirus 2 (SARS‐CoV‐2 in magenta), and for human Prohibitin in red. It can be observed that corresponding phases are almost opposite (195.5o or 1.08π rad), suggesting protein interaction.
In Table 1, found interactions between studied human and viral proteins are represented.
Discussion
This study revealed the presence of two consensus frequencies present in both SARS CoV replicases and methyl transferases, f1 at 0.2839 and f2 at 0.07349. Frequency f2 is common for interactions with prohibitins as well as CD4 T receptors, phase analysis suggests that SARSCov2 methyl transferase binds to human prohibitin, but not to CD4 receptors, whereas SARS Cov2 replicase binds to membrane CD4 T receptor. Binding of replicase to PHB takes place at other frequency, namely f3=0.0318.
These results might reveal certain implications. Thus f2, corresponding to an infrared wave at 2735 nm is expected to play an important role in the interaction between viral and human proteins.
Our results seem to be compatible with known facts about the four proteins studied.
During coronavirus infection, replication starts with the human cell penetration. At this stage, the virus losses its cap and viral RNA is released into the cytoplasm. Viral RNA has a methylated cap, and this helps disguising in such a way that host cell cannot identify it as alien, making it possible the binding to ribosome and subsequent translation 16. The first protein synthesized by host cell ribosome is the replicase.17) Viral proteases cleave the replicase at several sites, originating several structural, and 16 nonstructural proteins (NSP’s). The last of them, NSP16, corresponds to 2'-O-methyltransferase. Methyltransferase plays a role in cap methylation and, correspondingly is regarded as a major target in disease therapy. Being a sequence contained in the native replicase, it is not surprising the existence of shared frequencies, as well as shared interaction with prohibitins. Being the first protein to be synthesized by the host cell, it seems plausible to observe viral replicase interaction with human CD4 T cell receptors.
Prohibitin plays a key role in viral infection. Thus, it has been shown that PHB is a receptor mediating dengue virus DENV-2entry into insect cells. 18-20
It has been reported that PHB binds to several SARS CoV NSP’s 16 and binding to nsp16 would not be surprising. However, this seems to be the first evidence from literature supporting this interaction.
Thus, the Resonant Recognition Model is again providing concrete evidences for the interaction between SARS Cov2 proteins and proteins from human cells. Prospectively, this could help in the design of new peptides that could help in the production of new vaccines as well as the possibility to explore the use of coherent red light at 2735nm as a way to curb viral infection among certain groups of patients. The idea is not new 10), (12), (14, but never has been tested so far.
Conclusions
Application of Resonant Recognition Model to the study of coronaviruses revealed common RRM frequencies for both replicases and methyl transferases, and added plausibility to interactions between SARSCoV2 methyl transferase and human prohibitin, as well as between SARS Cov2 replicase and human prohibitin and CD4 T-cell receptors.