Introduction
As a watershed, penicillin discovery by Alexander Fleming in 1928, brought along the birth of the antibiotics era. Across the twentieth century, increasingly powerful drugs, both natural and synthetic, began to dominate the field of pharmacological treatment of infectious diseases, mainly caused by microorganisms, whether they were protozoa, bacteria or fungi. Mycoses considerably contribute to human morbidity and mortality, especially in immunocompromised patients.1 Although invasive fungal infections have a lower incidence, they are associated with higher mortality rates than tuberculosis or malaria, since about 300 million people suffer from some systemic mycosis, and their victims are annually accounted by millions.2
Cryptococcal meningitis is one of the most significant systemic mycoses, becoming the third most prevalent disease in patients with HIV/AIDS. Manifestations of cryptococcal infections range from limited skin infections to highly fatal systemic ones. Its incidence is quite high among the millions of seropositives; recent estimates points to one million cases of cryptococcal meningitis per year globally in patients with Acquired Immune Deficiency Syndrome. In sub-Saharan Africa, a region where HIV/AIDS is a relentless scourge, this disease claims more than 180 000 lives of HIV-positive patients every year.3 Due to financial constraints on accessing first-line antifungal medications, and increasing rates of resistance to them, one-year mortality for Cryptococcus related meningoencephalitis in HIV-infected patients varies from 50-100% in poor regions, compared to 10-30 % in rich countries.4
Even though the last seven decades have witnessed considerable progress in research of new antifungals, this progress has always been shadowed by drug resistance mechanisms developed by pathogens, thus generating a growing need for novel agents capable of reduce these harmful organisms. The most typical cases of resistance are related to azole derivatives, which are used both for human and animal health, in agriculture, in the wood industry, among other uses. The ubiquity of this class of compounds, due their multiplicity of uses, has accelerated the independent evolution of resistance in several environments.5 If this threat were not plain enough, at the very beginning of COVID-19 pandemic emergency in 2020, cases of infection by invasive fungal diseases were detected in patients with SARS-Cov2, in Nanjing, China, including C. neoformans var. grubii, recognized as aetiological agent of cryptococcal disease.6
Fluconazol and voriconazole resistant strains of C. neoformans have appeared in recent years, raising serious concerns once again, about the lack of effective treatments.3 Enzymes from sterol biosynthesis pathway, encoded by genes identified as erg(1-27), are the molecular structures mostly targeted by antifungals; among them, lanosterol 14α-demethylase or CYP51 (EC 1.14.13.70), catalyzes the oxidative removal of methyl group found in position 14α of lanosterol molecule, which is the first step for the biosynthesis of ergosterol and has been a major target for the pathogens of Fungi kingdom during decades.7 Stabilization of the complex CYP51-inhibitor depends on the interactions of the latter with amino acid residues within the catalytic site of the enzyme, in consequence, the genesis of drug resistance through this mechanism lies on the acquisition of point mutations in erg11 gene.7
Nowadays, tools of structural biology, bioinformatics and computational chemistry are unavoidably required, since the knowledge and exhaustive analysis of the structural features of macromolecular targets, are absolutely essential for development of new generations of inhibitors. The Protein Data Bank PDB (wwPDB) is the main provider of publicly available three-dimensional protein structures, resolved by X-ray crystallography, nuclear magnetic resonance and cryogenic transmission electron microscopy.8 Most of the resolved crystal structures of CYP51 are from bacterial organisms, but to date, crystallographic structures of CYP51 have been obtained only for four fungal species: Saccharomyces cerevisiae, Aspergillus fumigatus, Candida albicans and Candida glabrata.7 Comparative modelling using templates (homology modelling) is by far the most extended approach to predict the 3D structure of proteins, due its low computational cost and the possibility of obtaining models that are trustworthy enough to be used in further modelling studies, such as molecular docking or molecular dynamics.
Taking the above into consideration, the goal of present work is to obtain a homology model of lanosterol 14α-demethylase from Cryptococcus neoformans, reliable enough, such that inhibition modelling studies can be accomplished in later works. In silico structural characterization of the protein sequence and the predicted three-dimensional model, will be completed as well.
Materials and methods
The sequences were extracted from the Swissprot database in fasta format and the three-dimensional structures of templates from wwPDB in .pdb format.9 The sequence of the enzyme lanosterol 14α-demethylase from Cryptococcus neoformans var. grubii (serotype A) (CnCYP51) was cloned, expressed and reported in 2004 with the accession code Q870D1.10 Physico-chemical parameters were estimated with the ProtParam tool.11 Hydropathic and Transmembrane Tendency profiles for CnCYP51 sequence were constructed with ProtScale.11 Both ProtParam and ProtScale are implemented on the ExPASy server. The secondary structure of the protein was analyzed with PSIPRED server.12
Homology models were constructed with Modeller program (version 9.23).13 PSI-BLAST 14 and COBALT 15 were used for search and alignments. The UCSF Chimera software package (version 1.11.2) 16 was used as a graphic viewer for models and to prepare the structures. Screening of homologous crystal structures with at least 40 % of identity with CnCYP51 sequence was fulfilled, and all those proteins whose sequences satisfied that condition were downloaded in .pdb format, in order to be used as templates. Template structures were cleaned from non-standard residues (water molecules, ions, drugs or substrates); in case of heme cofactor, it was kept only in one structure (the lower resolution one) and deleted from the rest, in order to prevent its duplication.
Needleman-Wunsch dynamic programming algorithm was used, in order to obtain the multiple-template sequence alignment in .pir format, as input for Modeller. Once the alignment was done from the Chimera package interface, Modeller was executed to perform the calculation. The rest of the parameters were kept with their default values, to finally build five models. Once the calculation was completed, the model with the best value of DOPE parameter was selected for further refinement. Five refined structures were obtained for selected CnCYP51 model, through i3Drefine software (version 1.0)17; an all-atom homology model was provided as input. Scores from MolProbity and RWPlus programs, as well as 3Drefine score, were used in order to evaluate and compare the refined models.18
For scoring and evaluation of model quality the tools PROCHECK 19, ProSA 20 and Verify3D 21 were used. Ramachandran analysis was carried out with online PROCHECK, implemented in the European Bioinformatics Institute website. Global quality of the model was evaluated with ProSA (online version), through the construction of its pseudo-energetic profile. Three-dimensional profile of de protein model was calculated with Verify3D (online version).
For further characterization of CnCYP51 model, a profile of evolutionary conservation of amino acid positions in the sequence was obtained with ConSurf server.22 Computationally guided generation of site-directed amino acids mutations in CnCYP51 model was executed for the Y145F, G470R, and G484S substitutions, all of them responsible for fluconazole resistance. Mutant enzymes were obtained using the Dunbrack and Dynameomics rotamer libraries, both implemented in the UCSF Chimera package. The criteria for the selection of rotamers were based on the probability and the minimum number of steric collisions of the mutated side chains with the environment.
In order to improve the quality and distorted geometries of CnCYP51enzyme, as well as those of their mutant homologous models, the overall energy of the structures was minimized through the molecular dynamics routines provided by the MMTK (Molecular Modelling ToolKit) libraries. For standard residues, the parameters of 2014 updated version of the Amber force field (Amber ff14SB) were used, and the Antechamber module (also included in Chimera) is used to assign parameters to non-standard residues; semi-empirical method "Austin Model 1" with corrected binding charges (AM1-BCC), was used for the assignment of charges to non-peptide fragments (the heme group in this case). Steepest descent and conjugate gradient algorithms were applied sequencially; parameters were adjusted to 100 steps of the steepest descent method (step size = 0,02 Ǻ), followed by 10 steps of conjugate gradient (step size = 0,02 Ǻ). Interaction within the catalytic pocket were accounted with LigPlot+.23 Exploration of cavities and access tunnels to the active site was carried out with CAVER software (version 3.0.2).24
Results and discussion
Protein characterization from primary structure
Protein sequence characterization stands as an important analysis, since it might provide some basic information about protein structure and its properties, e.g., solubility, function.
Physico-chemical properties estimation
Physicochemical properties are criteria that allow assessing functional and structural characteristics of a protein. Predicted parameters were atomic composition, theoretical isoelectric point (pI), aliphatic index, extinction coefficients (ξ), estimated half-life in vivo and grand average of hydropathicity (table 1).
Table 1 Parameters obtained for CnCYP51 sequence by ProtParam.
Parameters | CnCYP51 | Parameters | CnCYP51 |
---|---|---|---|
Molecular Formula | C2829H4366N736O801S25Fe | ξa / Abs | 706 25 L·mol-1·cm-1 / 1.144 |
Molecular Weight | 61 745,09 Da | ξb / Abs | 702 50 L·mol-1·cm-1 / 1.138 |
Theoretical pI | 8,00 | Estimated half-life ( |
> 20 hours |
Aliphatic Index | 82,72 | Grand average of hydropathicity (GRAVY) | -0,248 |
a: ξ value assuming all Cys pairs in cystine form.
b: ξ value assuming all Cys pairs in -SH form. All ξ and absorbance values are calculated for λ = 280 nm and ρ protein = 1 g·L-1.
The molecular weight value provided by ProtParam is determined by adding the average values of the isotopic masses of amino acids of the protein and the average isotopic mass of a water molecule. The pI accounts for the pH value at which the overall charge state of a protein reaches neutral charge state.25 This parameter is critical in separation-purification techniques (e.g. electrophoresis and ion-exchange chromatography) since a zero-charged protein does not migrate under the effect of an electric field. Theoretical pI for CnCYP51 is estimated in 8, 00, indicative of a positive charged protein, predicting it to be basic in nature.
The aliphatic index of a protein can be defined as the relative volume occupied by the aliphatic side chains of the amino acids alanine, valine, isoleucine and leucine. In case of CnCYP51 it could be said that it has 82, 72 percent of aliphatic character. According to Guo et al. the aliphatic index is considered to be positively correlated with thermostability of globular proteins 26, thus, it suggests that CnCYP51 might be a thermostable enzyme. ProtParam offers two values for molar extinction coefficient, both for proteins in aqueous solution, measured at 280 nm. Software estimates ξ for a protein, just starting from the knowledge of its amino acid composition. The first one is based on the assumption that all cysteine residues are forming disulfide bonds, whereas the second assumes that all cysteine residues are reduced. In vivo half-life is a prediction of the time it takes for half the amount of protein in a cell to disappear after being synthesized in the cell. This value for CnCYP51 exceeds the 20 hours. The ProtParam criterion is based on the “N-terminal rule”, which relates the half-life of a protein with the identity of its N-terminal residue; in this case the estimate is given for a yeast in general.
Hydropathicity scales are values that define the relative hydrophobicity or hydrophilicity of amino acid residues. This feature is for the protein structure since, in general, hydrophobic amino acids tend to fold towards the interior of the protein, whereas hydrophilic amino acids are more frequently found on the surface of the macromolecule. The so-called hydropathy index is calculated from the Kyte-Doolittle scale, by summing the hydropathy values of all the amino acids of the sequence divided by the total number of residues. The higher the number, the higher lipophilic character of the amino acid; the most hydrophobic amino acids are isoleucine (4,5) and valine (4,2); the most hydrophilic are arginine (-4,5) and lysine (-3.9). ProtParam tool offers this parameter through the GRAVY value. For the enzyme CnCYP51 GRAVY = -0,248.
However, a more interesting result is obtained by plotting the scale values for all amino acids in the protein, as shown in figure 1. Commonly this lipophilicity/hydrophilicity criterion is used to predict which of the alpha helixes belong to transmembrane domains in protein. When plotting consecutively the values of hydropathy scale for each amino acids of the protein, variations around positive values indicate specific protein regions that show affinity towards the lipophilic interior of the lipid bilayer.
In CnCYP51 the N-terminal region displays positive values, which is consistent with the profile that shows the tendency of the sequence to form transmembrane structures, also represented in the graphs. Both profiles were computed with ProtScale tool. Although transmembrane domains must be embedded in the lipid bilayer, it is noteworthy that the former do not show a pronounced hydrophobic character, as might be thought; according to Bogdanov et al.27, even though lipophilicity is an important criterion for insertion efficiency within a membrane, studies applied to large-scale genomic data reveal that transmembrane domains tend to exhibit a “semi-hydrophobic” character.
Analysis of the secondary structure
Figure 2 shows the 2D representations of the secondary structure, obtained through PSIPRED server. PSIPRED is a highly accurate secondary structure prediction method that incorporates two feed-forward neural networks that carry out an analysis of the output obtained from PSI-BLAST, that is, it first executes an alignment with sequences that share structural similarity.12
This approach also applies a very strict cross-validation method to evaluate the performance of the method. As can be seen, structural motifs of alpha helices are predominant, built by 46, 80 % of total residues. The beta sheet structures are minority in the enzyme, reaching only 10, 70 %; the rest of the secondary structure is formed mainly by turns and loop-type structural motifs.
Search and alignment of homologous sequences of CnCYP51
Starting amino acid sequence of CnCYP51, a search for homologous proteins that shared an identity (i.e., similarity) of at least 40 % within the wwPDB was carried out with the PSI-BLAST tool. By having a template with more than 40 % identity to the target protein, the model is likely to have around 90 % of the modelled backbone atoms with a root mean square deviation (RMSD) of around 1Å regarding the crystallographic structure, largely due the probability that the templates might be similar to X-ray structure of target protein is very high. This deviation is comparable to the global differences between structures obtained, for the same protein, by NMR (already refined) and by X-ray crystallography.28
When searching for experimental fungal CYP51 structures, we found that only a few are available. In 2014 Monk et al. reported the crystalline structure of CYP51 from the harmless yeast Saccharomyces cerevisiae (brewer’s yeast); but only until late 2015 it was possible to obtain the first experimental CYP51 for a human pathogen species (from Aspergillus fumigatus, CYP51B).7 Table 2 shows the structures that were taken from wwPDB as templates to model CnCYP51. The structures found were ordered according to the percentage of identity. As can be seen, from Aspergillus fumigatus and Candida albicans, two crystalline structures of each species were taken; This was due to the fact that, in the case of C. albicans, they are two different strains of the species, where the erg11 gene that encodes the biosynthesis of the protein has mutated, resulting in the expression of two different protein sequences.
Table 2 Crystal structures used as templates to build CnCYP51 homology model.
No. | PDB Code | Identity (%) | Resolution (Å) | Organism |
1 | 4UYL | 49,3 | 2,55 |
|
2 | 6CR2 | 49,2 | 2,38 | |
3 | 5JLC | 43,9 | 2,40 | |
4 | 5FSA | 47,8 | 2,00 | |
5 | 5V5Z | 46,0 | 2,90 |
|
6 | 4LXJ | 46,1 | 1,90 |
In the case of A. fumigatus, even though it is the same strain and, therefore, there are no differences in the primary structures of both enzymes, the protein with identifier PDB 4UYL is complexed with the drug voriconazole (an antifungal similar to fluconazole), whereas drug VNI (an antiprotozoal used for the treatment of Chagas disease) binds the second (6CR2). Both structures were included in order to take advantage of their differences, since different ligands produce dissimilar conformations of the protein. Two theoretical models might explain the latter assertion: the induced fit model 29 and the conformational selection model 30; regardless of the mechanism that takes place to a greater or lesser extent, the unequivocal result is that there are two different templates, since their structures are not superimposable, even they have the same sequence.
Homology modelling
The most representative model is the one with the lowest value of the DOPE parameter. DOPE (Discrete Optimized Protein Energy) is an atomic distance dependent statistical potential derived from a sample of 1472 crystallographic structures and is completely based on probability theory. DOPE values for the five models ranged between -0,297 and -0,387. Although Modeller allows to obtain up to 1000 models, only five were built in order to optimize the calculation, since significantly better results would not really be achieved if a greater number were generated 28 and this would only make the whole process more computationally expensive. The best value, that is, the most negative, of the DOPE potential is possessed by model 2 (DOPE = -0,387). This was then the one chosen to be refined in the next stage.
Refinement of the model
Default configuration of i3Drefine program allows to generate five possible models; the best of them was selected from the different scoring functions. Contact analysis of all the atoms is the central feature of MolProbity score, which is achieved by adding and optimizing all the hydrogen atoms and then calculating their interactions, steric clashes and favourable contacts of the van der Waals radii.18 The output number reflects the expected crystallographic resolution for those values, so the best are the lowest. On its side, RWPlus was developed on the basis of a function of statistical atomic potential dependent on pairwise distance, using a chain of ideal “random walk” (mathematical formalization of a trajectory from a stochastic process in a succession of random steps in some mathematical space) as a reference state, to explain the connectivity of the sequence and the entropic elasticity of the proteins 18; more negative values generally indicate better quality. The 3Drefine score is a criterion based on the potential energy of the refined model, according to the force field implemented in the software, where lower values indicate a better quality of the model. The best refined model for CnCYP51 presented structural improvements compared to original homology model, as will be seen further on, in validation stage.
Model evaluation and validation
Figure 3 shows the Ramachandran Plots for CnCYP51 model before and after refinement. This type of diagram is one of the most used to establish how reliable a model is, whether it is an experimental structure or a theoretical model; it shows the torsion angles Φ-Ψ (phi-psi) for all residues in the structure (except those terminals of the chain). Glycine residues are identified separately by triangles, as they are not restricted to regions of the plot appropriate for the other types of side chains.
Ideally, it is expected that more than 90 % of the residues would be located in “core” regions. The ratio of residues in “core” (the red ones) regions is one of the best guides for stereochemical quality assessment of protein models. Summary of diagrams is illustrated through table 3. It is noticeable the increase in the number of residues with local torsions in the most favoured regions of the diagram, that took place after refinement. Even though a slight increase in the number of residues in disallowed regions of the diagram occurs, there was an overall improvement after the refinement step.
Evaluation of interactions of new inhibitors within the active site of fungal CYP51s, have been reported in the literature for several theoretical models.7 However, to our knowledge, only two models have been reported for three-dimensional structure of CnCYP51 prior to this work, both based on Mycobacterium tuberculosis CYP51 (MtCYP51). Comparison of the model obtained in present study with those obtained by Sheng et al.10 and Babazadeh-Qazijahani et al.31 on the basis of stereochemical analysis, would slightly tip the scales in favor of the former. Although occupancy degree in allowed regions yielded more than 95 % in all three cases (97,5, 95,3 and 97,1, respectively), the quality of model proposed by Sheng et al. is lower, since just 83,0 % of residues are located in the most favoured regions, compared to 90,5 % in two other models. It might be a consequence of identity between sequences of target structure and template, which is around 29 %. When using a simple alignment of two sequences with identity less than 30 %, the quality and reliability of the model obtained will tend to be low.28
Table 3 Distribution of residues in Ramachandran Plots before and after refinement.
Φ-Ψ Regions | Before refinement | After refinement |
---|---|---|
Residues in most favoured regions | 87,08 | 90,47 |
Residues in additional allowed regions | 10,17 | 6,99 |
Residues in generously allowed regions | 1,48 | 1,06 |
Residues in disallowed regions | 1,27 | 1,48 |
On the other hand, Ramachandran analysis is similar for the two remaining models, even when Babazadeh-Qazijahani et al. used as template the same MtCYP51 crystal structure, meanwhile our model uses an alignment of multiple templates, with identities above 40 %, each. The answer to this issue might underlie in the first portion of the sequence, which constitutes the transmembrane domain of the protein. The regions that function as anchors embedded in lipid membranes are essentially made up of alpha helices and are often regions that are difficult to resolve and refine; MtCYP51 lacks this type of domain, so the first 57 amino acids of the chain, belonging to N-terminal region of the chain, were eliminated from CnCYP51 sequence in order to improve the alignment. The model obtained in the present study, however, did conserve the transmembrane domain, which (as will be seen later in further analysis) will result in the region with the highest potential energy of the entire chain. Disregarding this fragment of the sequence might have contributed to obtain a more stereochemically “adequate” model by these researchers, however, in CYP51 structures the transmembrane helix is closely related to the catalytic domain, and influences the orientation it adopts, as well as the characteristics of the possible input tunnels of the substrate and output of the product. 7 For this reason, truncation of this segment of the sequence would not allow the most accurate description of the active site.
Global g-factor of the structure
Another criterion implemented in PROCHECK to assess the general quality of a model is the global geometry factor (overall g-factor), which is a measure of the normality, in statistical terms, of a structure. Global g- factor values provided by PROCHECK are typically between 0 and -0,5, where the best are those closest to 0.19 For the initially built structure these values is not at all favourable (-1,04), perhaps as a result of geometric distortions in the model. In contrast, the value for the best refined model of CnCYP51 was -0,08, which allow to consider the refined model as reliable.
Energy analysis
ProSA bioinformatic tool has a scoring function, which is derived from empirical information, under the assumption that the distances between two types of amino acids in different structures hosted in the wwPDB follow a Boltzmann distribution (figure 4).
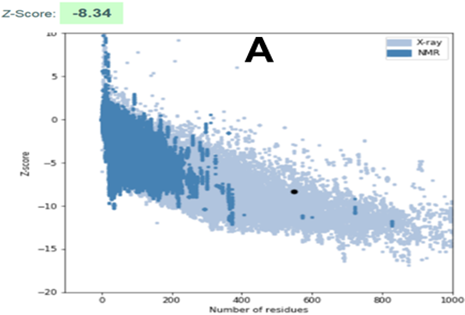
Fig. 4 ProSA analysis. A: Z-score scatter plot; dark dot represents CnCYP51 model. B: pseudo-energy profile for CnCYP51 model.
The Z-score of Pair Potential (expressed in kcal·mol-1) can be defined as the energetic separation between the native conformation of the protein (experimental) and the mean of a subset of the conformational space of the macromolecule, normalized by the standard deviation of this value.20 There it is the genesis of the term “statistical potential”, also widely used to denote the ProSA Z-score.
Figure 4A shows the plots of Z-score values for all native-like protein structures hosted in the current wwPDB repository, as a function of protein size. Results of the analysis are appreciated, both before and after refinement, through a dark dot in figure. It is observed that the modelled structure of CnCYP51 is located in the region expected for a model considered of good quality. It should be noticed that there was a slight improvement in the score after refinement; and the final model is considered reliable since those values outside the range of the scatter plot are considered to come from erroneous structures. Unreliable regions of the model are appraised through calculation of a pseudo-energy profile which reports weighted values f the potential energy as a function of the position of the amino acids in the chain. Inverse Boltzmann distribution is used to derive those pseudo-energy values, starting from a non-redundant set of experimental structures.32
In figure 4B the profile built for the best refined structure can be observed. Along the chain (starting from the N-terminal region) the interaction energy presents values below zero for most of the residues, which confirms the consistency of the theoretical model. The residues with higher positive interaction energies are located, mainly at the N-terminal region of the protein. The fact that in two templates (AfCYP51B and CaCYP51) transmembrane domain is not well resolved might explain this.
3D-1D Profile
The 3D-1D profile of a protein structure measures how compatible is a protein model regarding its own sequence, by assigning a structural class based on the location and environment of each residue, and comparing the results with experimentally validated structures.21 3D-1D profile for CnCYP51 homology model can be appraised in figure 5.
In Verify3D the profile is represented in the form of a plot of the average 3D-1D local scores in a 21-residue window; values may vary from worst (-1) to better (+1) scores, and correctly modelled structures exhibit better correlations and consequently, higher scores. In contrast, three-dimensional profiles computed from known incorrect protein models have a low score.32 To consider the atomic model and its own primary structure to be compatible, the model must have more than 80 % of the 3D-1D correlation distribution per residue with a score ≥ 0,2. As can be seen, 84,83 % of the residues, on average, have reached that value, so this analysis supports the reliability of the model. The N-terminal region is the region with scores lower than 0.2, which is consistent with previous energy analysis.
Characterization of three-dimensional structure
Three-dimensional structure analysis allows to obtain a better description of the enzyme catalytic region, by accounting for, e.g, access routes for small chemical species, or supramolecular interactions between residues, prosthetic groups and other molecules.
Interactions of heme ring within the active site of CnCYP51
As in all metallo-enzymes from cytochrome P450 complex, the region around the heme group occupies a central place in the function of the lanosterol 14α-demethylase from C. neoformans, since it encompasses the active site of the enzyme. In figure 6 the interactions between the heme cofactor and the surrounding residues are schematically plotted in two dimensions, by Ligplot+, pointing that in CnCYP51 the heme group is bound to the active site of the enzyme by supramolecular interactions (i.e., hydrophobic, van der Waals type and hydrogen bonds).
In our theoretical model the protoporphyrin ring system as well as the alkyl side chains, are found interacting with the environmental hydrophobic residues, e.g., Leu152, Lys156, Leu314, Ala317, Ile389, Phe483, Gly484, Val491 and Gly492. Within the cysteine pocket, Cys490 plays the role of thiolate ligand, through a coordination bond with the Fe3+ cation, from an axial position regarding to the plane of the ring. Tyr145, Arg391 and His488 form a network of hydrogen bonds with the cofactor to confine it deep within the catalytic cavity of the protein.
Cavity analysis and access routes to the active site
Proteins are highly complex systems, since they have asymmetric and irregular molecular surfaces, and on this they usually contain several clefts, protrusions and empty spaces. These internal openings might have specific functions, either as active sites, or as tunnels that could serve as transport routes for small size molecules (e.g., ions, water molecules, substrates, cofactors or other ligands, drugs).33 However, the experimental techniques for the study of access routes and diffusion processes of ligands into the enzymes are still unaffordable for most laboratories and researchers. Hence, in silico methods have become a matter of routine in the study of the cavities and their functions in globular proteins, and it is already an area of research in full development.33
Two main tunnels were described, with 11,7 Å and 13,7 Å in length, whose shape is formed by twenty-five residues, each. The second, although longer, has a narrower entrance, and a “bottleneck” at 8,8 Å from the molecular surface, defined by the residues Asp237, Phe240, His320, Met528 and Ile529; there, inner radius narrows to just 1.0 Å. This passage would be too tight, even for the substrate itself, which leads us to think that this access must be destined for the entry of much smaller molecular species, i.e., water, ions, O2. Meanwhile, the other tunnel has its narrowest point at 10.3 Å from the molecular surface; it is outlined by ten residues (Tyr131, Leu134, Phe240, Phe245, Ile386, Ser388, Ile389, Tyr390, Met528 and Ile529), but its radius is 1,9 Å, so this would allow the passage of molecules as large as sterols or drugs.
Evolutionary conservation analysis of CnCYP51 sequence
In general, evolutionary information is a powerful tool in structural and functional studies of proteins; they are especially useful to identify residues with a significant functional weight. ConSurf software provides a precise calculation of evolutionary rates through an empirical method based on Bayesian inference.22 In figure 7, regions with a high degree of conservation are marked with a color code. It is the residues forming the catalytic center of CnCYP51.
Results are expressed in the form of a score of conservation of the residues, where the most negative values represent the most conserved positions, while the most positive represent a greater variability. Analysis of position conservation between members of the same family can often reveal the importance of each position, since a mutation that involves the substitution of an amino acid that fulfills a significant role (either for preserving the three-dimensional structure, or due the functions it might carry out, e.g., catalysis, electron transfer) has a very low probability of occurrence, since key amino acids are subject to strong constraints of evolutionary selection , which limits transmission of these variations to next generations.22
Inside these conserved regions there are a few signature motifs typically found in enzymes from cytochrome P450 complex, such as the EXXR motif (377ERLR382). The heme-binding region (HBR), built by the conserved sequence FXXGXXXCXGX (482FGAGRHRCVGE494), is a conserved structural motif that, and along with the conserved threonine involved in proton
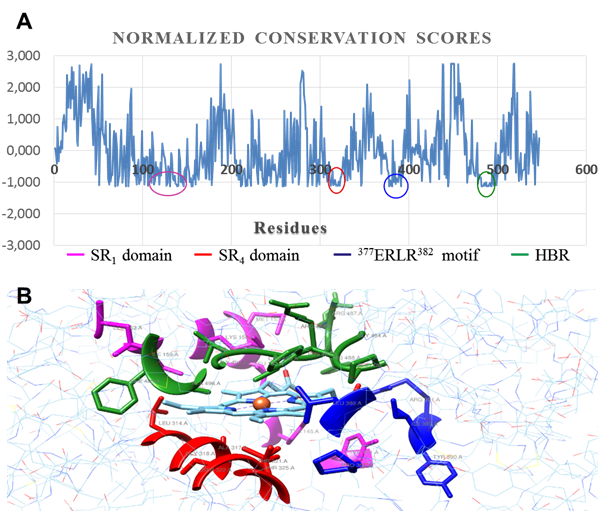
Fig. 7 Evolutionary conservation analysis. A: per residue conservation scores (normalized). B: catalytic pocket outlined by conserved regions
delivery (Thr321), it plays an active role in regulation of heme redox potential and the interactions with its redox partner to enable electron transfer to protoporphyrin macrocycle. The SRS1 and SRS4 domains (substrate recognition regions) are defined by sequences with relative conservation grade in CYP51 across Fungi kingdom. SRS1 motif defines the upper surface of the cavity where substrate is bound, whereas SRS4 shapes the right wall of distal surface of the cavity.34
Computer-guided amino acid mutation in the enzyme CnCYP51
After minimizing the protein structures, a comparison was established between CnCYP51 and its mutant homologues, based on the root mean square deviation (RMSD), excluding mutated points. RMSD value is commonly used as a quantitative measure of similarity between two or more protein structures, and this makes sense only if their sequences are identical or nearly identical; hence it is used to evaluate the conformational change of polypeptide structures. A higher value of this parameter, accounts for deeper conformational changes in the chain.
Generally, mutations of the erg11 gene that generate resistance to drugs (typically azoles) are verified, either by blocking the access of the inhibitor to the catalytic center of the protein, or by reducing its interactions with residues close to the heme group, in such way that contact with the Fe3+ cation be noticeably limited. Y145F mutation, was first reported by Sionov et al. in 2012.35 The only structural difference between the amino acids tyrosine and phenylalanine is the phenolic hydroxyl group (‒OH) of the former, however, the RMSD value between the catalytic regions of both proteins is 3.84 Å. In a glance at another homologous CYP51 structure 36 from Saccharomyces cerevisiae (ScCYP51), complexed with fluconazole at the active site (PDB code: 4WMZ), which lacks this mutation, it is remarkable the role that tyrosine residue (Tyr140) plays in the orientation of the heme group. Meantime, it also contributes to the stabilization of fluconazole within the catalytic pocket of the enzyme, through the formation of a water bridge. In CnCYP51 Y145F causes a change in the polarity of the residue and eliminates the possibility of the aforementioned interaction. This would force the heme cofactor to subtly reorient itself (which is, however, perceivable in its effects), due to the axial traction effect over the plane of the heme ring, generated by the coordination bond between Cys490 and the ferric nucleus, thereby explaining the RMSD value.
Change from glycine to arginine at position 470 of the CnCYP51 chain is mediated by the G1785C mutation in the erg11 gene.35 This substitution takes place in a loop of the chain further away from catalytic, however, the side chain of Arg470, with an elongation of 7,54 Å from the C-α, can interact with the alpha helix built by sequence 492EQFAYTQLSTIFTYVVRN511. In the initial fragment of this helix formed by residues Val491, Gly492 and Glu493, there is a more pronounced torsion regarding to non-mutated structure, an effect that could also be reflected in Cys490, whose coordinated bond with Fe3+ serves as a pivot to heme position. The RMSD = 7,92 Å in the region adjacent to the cofactor, evidences the change in orientation as a direct effect of the G470R mutation.
Mutation G1885T at erg11 gene is expressed by the substitution of glycine by serine at position 484 35, by virtue of which side chain of Ser484 would interact with one of the carboxylate anions (RCOO-) of heme group, through hydrogen bonding. As a consequence of this interaction, higher restrictions would be imposed on the Csp3-Csp3 rotation between the C-α and the side chain of Ser484, and consequently to conformational space of the region defined by the sequence 465DYGFGSVSKGTESPYQPFGAGRHRCVG493; notice that the known as “cysteine pocket” (from Gln481 to Gly492) is a constituent portion of the fragment at issue. As a consequence of the latter, in order to find a lower potential energy state heme ring would withdraw deep towards the interior of the structure, making the environment of the cofactor even more lipophilic. Mutation G484S was also identified in 2009 by Sheng et al.10; they proposed a possible mechanism to elucidate how the amino acid substitution would cause an increase in the minimum inhibitory concentration of fluconazole in C. neoformans, which is consistent with our explanation, reflected in the RMSD value between the catalytic domains of both proteins (3,53 Å).
In general, the effect produced by all three mutations put under scrutiny in the present study has been a motion of the heme group deep inwards the structure. Thus, this finding ought to be reflected in the accessibility of azole inhibitors to the nucleus of the heme ring. A fairly reliable way to assess this would be to observe variations in the distance between the Fe3+ cation and the access tunnels to the catalytic center of the enzyme. The analysis of the cavities points out to heme group retracting into the structure in mutant enzymes, and also shows how distance between the tunnels and the heme group lengthened in three cases. While in the CnCYP51 enzyme the distance between the tunnels (measured from the center of the cross section of each tunnel) to the metal cation is 5,72 Å, in CnCYP51Y145F, CnCYP51G470R and CnCYP51G484S enzymes, distances are 8,70, 8,71 and 8,62 angstroms, respectively (figure 8).
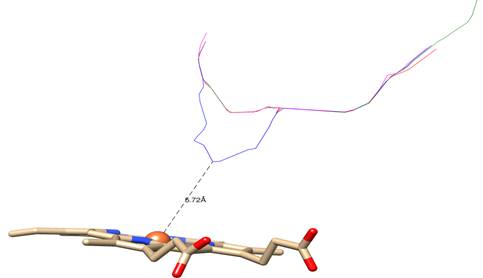
Fig. 8 Colored lines represent the centers of transversal sections of tunnels leading to catalytic site: blue: non-mutated CnCYP51; magenta: CnCYP51Y145F; green: CnCYP51G470R; red: CnCYP51G484S
The increase in the distance between the access tunnels to the active site and the heme cofactor could contribute to the explanation of the decreased sensitivity of C. neoformans strains with these erg11 gene mutations towards some drugs such as fluconazole and voriconazole since lanosterol molecule, unlike azole inhibitors, does not interact directly with the heme group.
Conclusions
A refined homology model was built for enzyme CYP51 from Cryptococcus neoformans. Ramachandran stereochemical analysis, yielding 97,46 % of residues located in the allowed regions; ProSA analysis, that placed the model within expected interval in Z-score scatter plot (Z-score = -8,34); as well as the distribution of 3D-1D correlation left 84,83 % of residues with average score ≥ 0,2, have serve as trials for quality assessment, which has confirmed the reliability of CnCYP51 model. However, further refinement of the structure could be achieved through molecular dynamics studies.
Besides, two main access tunnels towards active site were characterized, as well as catalytic pocket containing heme cofactor, whose interactions with surrounding residues points to a pronounced hydrophobic character of that region. Evolutionary analysis has revealed a high conservation in the residues forming the catalytic site. The generation of computational site-directed substitutions resembling three previously reported point mutations, allowed to gain an insight into azole-resistance mechanisms in C. neoformans species.