Introduction
The photovoltaic solar energy (PV) has experienced an accelerated increase compared to the previous year, despite the global economic crisis caused by Covid-19 [1]. Reports published by International Energy Agency and National Renewable Energy Laboratory’s in 2022 show that in first nine months of 2021 the capacity of 171 GW of photovoltaic systems installed globally throughout the world was reached, while for 2022 and 2023 it’s expected to reach 209 GW and 231 GW respectively [1], [2]. According to statistics report published by International Renewable Energy Agency in 2021, Cuba ended that year with 163 MW of installed capacity of photovotlaic systems [3]. The Ministry of Energy and Mines has the plan of reaching 24% penetration with renewable sources by 2030 [4].
For this reason, studies about the renewable energy sources have increased, such is the case of Díaz (2018), Gutiérrez et al., (2020); Gutiérrez et al., (2021a); Gutiérrez et al., (2021b), all the results have contributed to improving the efficiency of PV systems in the country [4], [5], [6], [7]. Even Korkiakoski (2021) and Alberto et al., (2021) studied the possibility of generating 100% energy from renewable sources by 2030, the Isla de la Juventud was taken as case study; demonstrating that’s possible to achieve it [8], [9]. The weather conditions in Cuba are very good for the development of solar energy, however, it’s a tropical island where the ambient temperature is higher than other regions of the world. Temperature is the climate variable that most affects the efficiency of the PV module, after irradiance [10]. Conventional photovoltaic cells are made of semiconductor material that produces electrical energy from the solar radiation that reaches it’s surface. In the process of converting from one type of energy to another, there are losses associated with various factors such as: production technology, shading, module temperature, angular losses, inverter losses, and cable losses. These parameters cause losses of 10-20%, being those that most affect the efficiency of photovoltaic conversion [11], [12].
Many investigations have correlated the efficiency with the operating temperature, the PV cell only converts a small amount of the irradiance it receives into electrical energy, the rest is dissipated as heat [13]. The electrical efficiency ηc is expressed as a function of the cell temperature Tc through the linear relationship shown in equation (1), [14]. This correlation has been widely accepted and for this reason it appears in experimental investigations for different environmental conditions [15], [16].
Where n STC (%) and β STC (%/°C) are the electrical efficiency and temperature coefficient of the cell, respectively. Tc (°C) and T STC (°C) are the cell operating temperature and the reference temperature, respectively. The n STC , β STC and T STC parameters are always provided at the PV module technical datasheet. The operating temperature Tc is a variable that is not commonly measured, but can be calculated by applying mathematical models that correlate some weather variables. Current models mainly correlate three climate variables: ambient temperature, irradiance and wind speed. These models are classified into three groups: constant temperature models, physical models and statistical models [17]. Some of these models do not use wind speed, while others do, the latter being the ones that have shown better accuracy in most of the studied cases [18].
Literature review: PV cell operating temperature estimate models
Constant temperature models use a constant value of the photovoltaic cells operating temperature to estimate the energy delivered for specific conditions of PV system. This type of model has the advantage that it can be used quickly to predict the performance of PV systems, since manufacturers always include the necessary parameters at the technical datasheet. The most used are the Standard Measurement Conditions (STC) and the Nominal Operating Cell Temperature (NOCT) [17], [19]. STC are specific laboratory conditions, they are defined as: irradiance G=1000 W/m2, PV cell temperature Tc=25 ºC and air mass AM=1.5 G [10], [20]. However, STC can be very different from the operating conditions of PV modules in a real environment in many places around the world. As alternative to the STC, Duffie & Beckman (2013) propose a model for NOCT conditions, defined by: G=800 W/m2, Tc=20 ºC and v=1 m/s [19], [21], [22]. These two models have the disadvantage that the proposed environmental conditions rarely coincide with the actual operating conditions of the PV systems.
Physical models have been established to calculate the operating temperature of modules dynamically, studies by Lawrence Kamuyu et al. (2018), Nguyen et al. (2021) and Sredenšek et al. (2021) are current evidence of these research topics, each one models Tc according to it's PV technology and it's geographical installation site, respectively [18], [23], [24]. In addition to irradiance and ambient temperature, some models also consider wind speed King et al. (2004), Faiman et al. (2008) and Koel et al. (2011) [25], [26], [27]; while others do not consider it, such as Akhsassi et al.[28]; but all agree that irradiance is the main cause that causes an increase in temperature of the PV cell. Statistical models can be subdivided into two groups: Artificial Intelligence (AI) methods and linear models. Studies that include AI to predict Tc consist of neural networks, soft computing, and adaptive neuro fuzzy inference system [29], [30], [31], [32].
These methods have the advantage of being very versatile, due to their ability to process complex trends; but as they are black box models where do not explicitly it show the relationship between the model variables. Linear statistical models are much simpler, some of the most applied in published research are Risser & Fuentes (1983) and Muzathik (2014) [33], [34]. These models correlate the module temperature (Tc) with the three main variables (G, Ta, v) in situ measured, but could be inaccurate when applied to any other site, for this reason these models need to be verified. The aforementioned studies predict the Tc from the radiation that falls on the surface of the PV cells and other measured climate variables, however, a general model that can satisfy all PV technologies has not been established. For example, Zouine et al. (2021) analyzes 48 combinations of models of the thermal and electrical behavior of PV cells of crystalline technologies, showing the diversity and relevance of this type of research [16].
Research aim
This research analyzes different established mathematical models, the aim is determinate the model that best correlation with Tc measurements of monocrystalline silicon PV modules, installed at the Solar Energy Research Center (CIES spanish acronym) in Santiago de Cuba. For the assessment, ten models widely referenced and recommended by the scientific community have been chosen. The variables that correlate are: ambient temperature (Ta), irradiance (G) and wind speed (v).
Also it's calculates the variation of the PV efficiency as a consequence of the behavior of the local climate variables, the results are useful to improve the reliability of the sizing of the PV systems for future projects to be developed in Cuba.
Materials and methods
Geolocation of PV system and instrumentation used in data monitoring
The climate data were measured at the CIES in Santiago de Cuba, a province located south of eastern Cuba. Specifically, it’s located at the geographic coordinates 20° 00′ 14″ N and 75° 46′ 10″ W, at 90.40 meters above sea level. Figure 1, shows the geolocation, the PV system and the weather station.
The setup of the photovoltaic generator installed in the CIES is presented in table 1, consists of 30 modules installed on the ground, grouped by 3 strings of 10 modules.
Table 1 Setup of PV system
Type of structure | Mounted on an aluminum base, with wind circulation. |
---|---|
Tilt angle | 15° |
Azimuth angle | 0° |
PV power | 7.5 kW |
The electrical parameters of the PV module are presented in table 2, its permissible operating temperature is -40 ºC to 80 ºC, these parameters are available in the technical datasheet.
Table 2 Electrical parameters of HELIENE215MA PV module [36]
Parameter | STC | NOCT |
---|---|---|
Peak Power Watts (Pmax) | 250 W | 183 W |
Open Circuit Voltage (Voc) | 37.40 V | 34.5 V |
Maximum Power Voltage (Vmpp) | 30.3 V | 27.7 V |
Short-Circuit Current (Isc) | 8.72 A | 7.25 A |
Maximum Power Currennt (Impp) | 8.22 A | 6.7 A |
Coefficient Temperature Voc (β) | -0.34 %/°C | - |
NOCT | - | 45 ºC |
Technology type | Monocrystalline | |
Efficiency | 15.03 % | |
Cells | 60 series connected cells |
The meteorological parameters including irradiance and ambient temperature were measured by sensors installed next to the PV system, while wind speed was measured by a weather station placed about the 10 meters above it. The module temperature sensor was adhered to the backside of a module, ensuring good contact. The technical details of sensors and the weather station are presented in table 3.
Table 3 Technical features of the measurement instruments
Components | Parameters | Accuracy | Model |
---|---|---|---|
Module’s temperature sensor | Tc (°C) | ±0.8 °C (in the range -20°C to 100°C) | PT1000 |
Weather Station | Ta (°C) | ±0.8 °C (in the range -40°C to 100°C) | METEODATA 3016 C |
G (W/m2) | ±5 % (average over a year) | ||
v (m/s) | 0.3 m/s |
The data measured includes the period from September 20 (2018) to March 31 (2019), with 10-minute sampling intervals, for a total of 27,648 measurements.
Assessed Tc estimation models
The table 4, shows 10 assessed models for the correlation of in situ measurements, including physical models, equations 2, 3, 4, 5, 6, 7, 8, and statistical models, equations 9, 10, 11. The considered models are widely known and applied in many study cases from various countries [24], [37], [38]. A few of them are the mathematical support of professional software, i.e. RETScreen, equation (2), PVsyst, equation (6) and System Advisor Model (SAM), equation (7), which are useful for the sizing and simulation of PV systems [15].
The STC and NOCT constant temperature models are not considered because it’s interesting to know the thermal performance of the modules for variable meteorological conditions. Note that equations 2, 3, 8 don’t use the wind for modeling.
Table 4 Models to estimate the module operating temperature. [14] , [22] , [25] , [26] , [27] , [33] , [34] , [39] , [40] , [41] , [42]
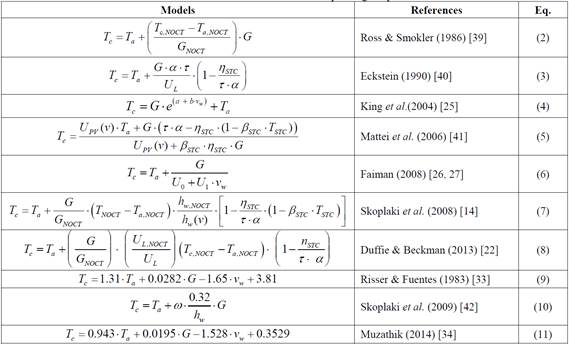
Where
T C |
the temperature of the PV cell (°C) |
Ta |
the ambient temperature (°C) |
T c,NOCT |
nominal operating temperature, depending on the manufacturing material it has a value of 44 °C to 46 °C |
T a,NOCT |
is ambient temperature for NOCT conditions, it has constant value of 20 °C |
G NOCT |
the irradiance for NOCT conditions, it has a constant value of G=800 W/m2 |
G |
the irradiance received at the receptor plane (W/m2) |
η STC |
the module efficiency for STC conditions |
τ·α |
the product of transmittance-absorbance, has a constant value of 0.9 [14] , [40] |
U PV |
the heat exchange coefficient at the surface of the module [40] , [41] |
β STC |
the coefficient of variation of voltage with temperature (%/°C) |
T STC |
the module operating temperature for STC conditions |
h W |
the wind convection coefficient (W·m-2·°C-1) |
h W,NOCT |
the convection coefficient for the wind in NOCT conditions (W·m-2·°C-1) |
v W |
the wind speed measured near the module (m/s) |
In equation (3), U L is the heat loss coefficient, a parameter that is calculated for each module by equation (12), [40]:
In equation (4), the coefficients a and b have been calculated, obtaining a=-3.473 and b=-0.0594, the main advantage is that it does not distinguish between different PV technologies [10], [43].
In equation (5), the parameter τ·α=0.81 while U PV is the heat exchange coefficient, it’s a linear function with the wind speed, it has two forms of parameterization represented by equations (13) and (14), to this equations are called as Mattei1 and Mattei2, respectively [41]:
In equation (6), for monocrystalline silicon technology, the constants U 0 =30.02 and U 1 =6.28 are used [27].
In equation (7) and (10), the parameter h W is the wind convection coefficient, it’s calculated by equation (15), [14].
In equation (8), the heat exchange coefficient is approximated by equation (16), [38].
In equation (10), the ω parameter is a mounting coefficient defined as the ratio between the Ross parameter for the mounting geometry and the Ross parameter for free-standing modules. It takes different values: 1 for free-standing, 1.2 for flat roof, 1.8 for sloped roof (well cooled) and 2.4 for facade integrated [10], [43]. By the setup of PV system of CIES at this study ω =1 is considered.
Statistical analysis for model assessment
In order to assessment the models, the coefficient of determination (R2), the root mean squared error (RMSE) and the mean absolute error (MAE) are calculated by equations 17, 18, 19, respectively [32], [38]. These statistical indicators help to determine the difference between the temperature simulated (Tc_sim) and the temperature measured (Tc_meas).
Results and discussion
The eastern region of Cuba, where the CIES of Santiago de Cuba is located, is characterized by high air temperatures throughout the year. On the left side of figure 2, it shows the histogram of the Ta measured, wich easily overcomes 30 °C; on the right side, it shows the histogram of Tc measured, which easily exceeds the reference values established on the technical datasheet.
A brief statistical summary of the climate variables is presented in table 5, the data were filtered to analyze only the measurements with the condition G > 0 W/m2. The filtered data removes also the night time measurements, that is, only registered measurements during daylight hours, between 6:00 to 18:00 hours were used.
Table 5 Statistical summary of the climate variables measured at CIES
Variable | Minimum | Mean | Maximum |
---|---|---|---|
Irradiance ( |
1 W/m2 | 513.96 W/m2 | 1247.00 W/m2 |
Wind speed ( |
0.08 m/s | 2.50 m/s | 8.49 m/s |
Ambient temperature ( |
20.00 °C | 29.39 °C | 35.00 °C |
Cell temperature ( |
17.00 °C | 41.98 °C | 67.00 °C |
Figure 3 and the summary of table 5, confirm that the weather conditions in Cuba, and in particular in Santiago de Cuba, are more higher than the STC conditions provided by the manufacturers technical datasheet of PV modules, in which the mean of Tc exceeds of reference (T=25 °C). Likewise, the maximum irradiance values exceed the reference value defined in technical datasheets. Hence the importance of applying the models to effectively predict Tc.
Analysis of the correlation of assessed models
The models in table 4, were correlated with the in situ measurements of Tc of the HELIENE215MA modules, applying equations 17, 18, 19 the statistical coefficients shown in table 6, were obtained. The best and worst correlation models are marked in bold.
Table 6 Statistical coefficients of the models
Models | R2 | RMSE (°C) | MAE (°C) |
---|---|---|---|
Ross & Smokler (1986) | 0.890 | 3.897 | 4.114 |
Eckstein (1990) | 0.894 | 3.835 | 3.012 |
King |
0.912 | 3.482 | 2.698 |
Mattei1 (2006) | 0.915 | 3.425 | 3.830 |
Mattei2 (2006) | 0.913 | 3.468 | 3.659 |
Faiman (2008) | 0.898 | 3.758 | 3.651 |
Skoplaki |
0.894 | 3.834 | 3.497 |
Duffie & Beckman (2013) | 0.803 | 5.216 | 5.817 |
Risser & Fuentes (1983) | 0.896 | 3.792 | 10.738 |
Skoplaki |
0.893 | 3.846 | 3.368 |
Muzathik (2014) | 0.881 | 4.060 | 8.091 |
According to the accuracy criterion of the coefficient of determination R2, the models with the best correlation are King et al. (2004) , the two variants Mattei (2006) and Skoplaki et al. (2009) with R2 > 91%; to a lesser extent are Ross & Smokler (1986), Eckstein (1990), Faiman (2008), Skoplaki et al. (2008) and Risser & Fuentes (1983), with R2 > 89%. The Duffie & Beckman (2013) model presented the worst correlation, with R2 < 82%.
The Ross & Smokler (1986) model does not use the wind, however, it has a better R2 coefficient than Duffie & Beckman (2013) model. The Eckstein (1990) model has a relationship with Tc that is practically the same as the Skoplaki et al. (2008), so similar R2 and RMSE results were obtained for both. All the models presented a high R2 correlation, which implies that any of these models could be used effectively for the estimation of Tc, but the Mattei1 (2006), Mattei2 (2006) and King et al. (2004) had very similar R2 and RMSE coefficients, so any of these three could be chosen to predict Tc. In order to decide which is the best correlationed model, the MAE coefficient was taken into account as the main accuracy criterion. Figure 3, shows a scatter plot with the relationship between RMSE and MAE coefficients, which help to make a final decision.
The figure 4, shows that the King et al. (2004), Mattei1 (2006) and Mattei2 (2006) models present a very small variation in terms of RMSE, but the King et al. (2004) is the model with better MAE accuracy, therefore, we considered that is the best choice to estimate Tc. For this reason, it’s recommended to use in sizing and economic feasibility studies for future photovoltaic system projects to be installed in the region.
Estimation of PV efficiency
To estimate the PV conversion efficiency of each model, the Tc values were substituted in equation 1. Figure 4, shows the linear correlation of the PV conversion efficiency with respect to Tc calculated from the model King et al. (2004). Note that the increase in temperatures has a decreasing effect on the efficiency of the PV modules, the points indicated in graph show that the efficiency decreased 1.9% for the time measured.
The worst model Risser & Fuentes (1983) returns maximum values of Tc=77.12 °C with MAE=10.74 °C, very far from the maximum measured Tc values shown in table 5. While King et al. (2004) is more sensitive to changes in operating temperature, the linear relationship of the temperature Tc with the efficiency ηc is indicated by figure 4. Therefore, it can be predicted the increase of 1 °C of temperature Tc has an approximate decreasing effect of -0.042 at ηc efficiency of the PV cell.
Simulation of Tc for variable weather conditions
To simulate the behavior of the best and lowest correlation models, two random days were chosen, clear sky and cloudy sky conditions, are presented in figures 5 and 6, respectively. On the left side, the graphs are from October 27, 2018 (clear sky), with regular solar radiation from sunrise to sunset, with a maximum value of G=902 W/m2, temperature in the range of 21 °C to 28 °C and wind speeds not plotted, with v=0.2 m/s (6:00 hours) - 2.26 m/s (18:00 hours) and a maximum value of 5.9 m/s (14:00 hours).
On the right side, the figure are from January 30, 2019 (cloudy sky), with irregular solar radiation during the hours of Sun, with a maximum value of G=1061 W/m2 at 11:00 hours, then it drops to the value of G=166 W/m2 at 13:30 hours, with the temperature in the range of 22 °C to 31 °C and wind speeds not plotted, with v=3.72 m/s (6:00 hours) - 1.12 m/s (18:00 hours) and a maximum value of 4.46 m/s (12:00 hours).
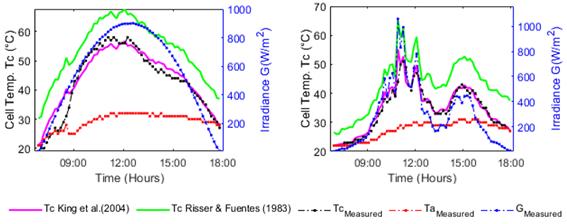
Fig. 5 Estimated Tc by the best and worst correlation model for clear sky (left) and cloudy sky (right) conditions
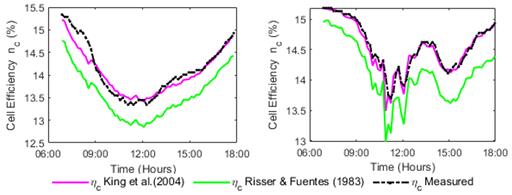
Fig. 6 Estimated efficiency ηc by the best and worst correlation model for clear sky (left) and cloudy sky (right) conditions
In both figures are verified that the irradiance G significantly influences the temperature of cell Tc, increasing and decreasing Tc according to the trend of G for each day represented. In the case of figure 6, it can be seen how the efficiency is higher the morning and the afternoon hours, reaching lower values in the midday hours, this is mainly due to the temperature of operation variation Tc. As the zenith approaches, the irradiance increases and consequently the operating temperature of the photovoltaic modules, which causes a maximum attenuation of efficiency ηc. This behavior is similar both on clear sky days and on cloudy days.
For the date October 27, 2018, the King et al. (2004) model has an efficiency at range of 15.18% - 13.49%, while Risser & Fuentes (1983) model was at range of 14.95%. - 12.98%. For the date January 30, 2019, the King et al. (2004) model has an efficiency at range of 15.23% - 13.41%, while the Risser & Fuentes (1983) model was at range of 14.76% - 12.85%. For both models, an energy efficiency value of PV modules was obtained below that established by the manufacturer at the technical datasheet, for clear sky conditions and also for cloudy sky conditions.
The statistical coefficients described above to assessment the two models are presented in table 7.
Table 7 Statistical coefficients of the best and worst correlation model
Models | Day October 27, 2018 (clear sky) | Day January 30, 2019 (cloudy sky) | ||||
---|---|---|---|---|---|---|
R2 | RMSE (°C) | MAE (°C) | R2 | RMSE (°C) | MAE (°C) | |
King |
0.947 | 2.672 | 2.447 | 0.943 | 1.862 | 1.219 |
Risser & Fuentes (1983) | 0.937 | 2.919 | 10.648 | 0.932 | 2.039 | 8.684 |
For analized days, the King et al. (2004) model had a better correlation than Risser & Fuentes (1983). The difference at MAE coefficient of both models is notable, while the coefficients of R2 and RMSE showed less difference.
Conclusions
The estimation of the operating temperature is very important for sizing and economic technical studies, because it improves the estimation of the energy efficiency of PV systems. The Tc measurements at the CIES in Santiago de Cuba are higher than the 25 °C defined in PV module technical datasheet, so it’s important to know the effect of Tc on the ηc of the PV cell. In this study, 10 models were assessed, from which it’s concluded:
The variables that correlated with the cell temperature Tc were the irradiance G, the ambient temperature Ta and the wind speed v, the King et al. (2004) model presented the best accuracy criteria, whit coefficientes R2=0.912, RMSE=3.482 °C and MAE=2.698 °C.
The King et al. (2004) model estimates the temperature Tc and it’s effect on the efficiency of the module, in such a way that the increase of 1 °C causes a decrease effect of -0.042 at the efficiency ηc of the PV cell. This linear relationship correctly estimates the energy behavior of the CIES modules for various weather conditions. For the months studied (September 2018 to March 2019), the efficiency dropped to 1.9% during the day, so in summer months it should decrease even more.
Although the study only includes monocrystalline silicon technology, in a period of time that does not cover the 12 months of year, the results are valid to know the effect of Tc on the efficiency of the PV modules installed in Santiago de Cuba.
According to the 2021 Statistical Yearbook of Cuba, the temperatures between provinces do not have a considerable difference, that why this study could be taken as a reference for any other region of the country for PV system projects [44]. As a future perspective, it’s planned to extend the study to other areas of Cuba and other PV technologies such as amorphous silicon and polycrystalline silicon, in order to establish a more accurate model that improves the estimate of Tc.